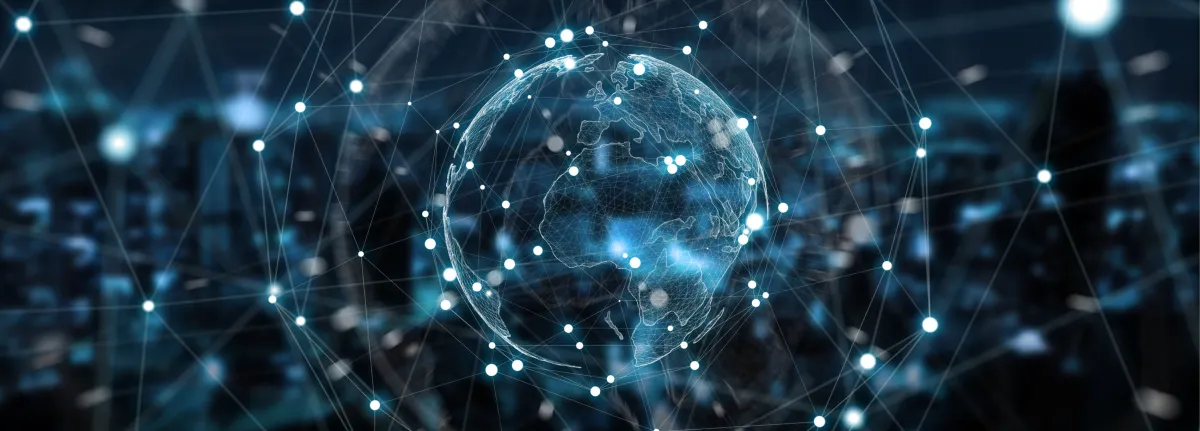
Callista AI Weekly (March 17 - 23)
The week of March 17–23, 2025 saw a surge of announcements and breakthroughs in artificial intelligence (AI). From innovative use cases in healthcare and robotics to the release of new AI models and agents, tech giants rolled out major product updates, and policymakers grappled with AI governance. Notably, Swiss institutions were active in shaping AI strategy and regulation. Below, we break down the most important developments of the week and their business implications for a Swiss audience.
1. New AI Use Cases
AI Enhancing Healthcare Delivery: Hospitals are leveraging AI to tackle staff shortages and improve patient safety. In the US, Emory Healthcare launched a “virtual nursing” program that combines telehealth and AI-driven fall-prevention technology (Emory Healthcare launches virtual nursing initiative using AI-driven technology to enhance patient care). At Emory University Hospital Midtown, remote “virtual nurses” handle routine tasks like admissions paperwork and patient discharge education via video links, freeing on-site nurses to focus on direct patient care. An AI platform by VirtuSense monitors patients’ rooms using spatial intelligence to predict and prevent falls, alerting staff before an incident occurs. For businesses in healthcare, this exemplifies how AI can augment the workforce and improve service quality. By automating time-consuming documentation and continuously monitoring patients, hospitals can reduce costs from preventable accidents and address nurse burnout—a critical benefit in an era of staffing challenges.
AI-Powered Home Robotics: The convergence of AI and robotics is opening new consumer markets. The Bot Company, a California robotics startup co-founded by former Cruise CEO Kyle Vogt, raised $150 million to develop AI-driven home robots (Exclusive: Former Cruise CEO Vogt's robotics startup valued at $2 billion in new funding, sources say | Reuters). This funding round valued the year-old company at $2 billion, reflecting investor excitement around robots that can perform household chores. The appeal lies in combining physical machines with AI models that learn new tasks. Advances in large language models (LLMs) have made robots more intuitive—enabling them to understand natural-language commands and execute complex tasks . For example, a home robot might use an LLM to interpret a voice request to “tidy the living room,” then coordinate its vacuuming and object-sorting functions accordingly. While The Bot Company’s product is not yet on the market, its rapid rise — along with humanoid robot ventures like Tesla’s Optimus and startup Figure (reportedly raising funds at a $40 billion valuation) — signals a coming wave of intelligent, adaptive robots. Businesses in manufacturing and home services should watch this trend closely: AI-driven automation could soon move from factories into everyday life, creating new opportunities (and competitors) in domestic services, elder care, and smart home integration.
AI in Customer Service and Retail: Companies are also deploying AI in customer-facing roles to boost engagement and sales. Adobe reported that consumers increasingly expect chatbot-style interactions on websites, similar to using ChatGPT (Adobe rolls out AI agents for online marketing tools | Reuters). In response, Adobe is helping brands add AI virtual agents to their digital marketing toolkit. For instance, a travel site can use an AI agent that greets visitors and provides personalized suggestions. If a user arrives via an Instagram ad for beach vacations and asks about trip bookings, the AI agent can cross-reference the ad content and live inventory to recommend relevant destinations. This level of personalization — tailoring responses based on the customer’s journey—can significantly improve conversion rates. Additionally, Adobe’s new tools let marketing teams instruct AI agents to optimize website layout or content to drive sales, changes that the AI can implement immediately. This drastically shortens website iteration cycles that previously took months of developer time. Meanwhile, in the fast-food industry, companies are piloting AI to streamline drive-thru operations. Yum! Brands (owner of Taco Bell) is reportedly partnering with Nvidia to leverage AI for more efficient drive-thrus, following similar trials by Wendy’s last year. The AI would dynamically suggest menu items and speed up order processing for customers. For Swiss retailers and consumer businesses, these cases illustrate AI’s potential to deliver more interactive, customized customer experiences and real-time optimization of sales channels.
2. Newly Launched or Updated Models and Agents
Nvidia’s “Llama Nemotron” Reasoning Models: At Nvidia’s GTC conference, the company unveiled a family of open AI models called Llama Nemotron, designed specifically for advanced reasoning tasks (NVIDIA Launches Family of Open Reasoning AI Models for Developers and Enterprises to Build Agentic AI Platforms | NVIDIA Newsroom). These models are built upon Meta’s Llama architecture but post-trained by Nvidia to excel at multi-step reasoning, math problem-solving, coding, and complex decision-making. Essentially, they provide a business-ready foundation for building AI agents that can work through problems step-by-step rather than just responding with a single-shot answer. Nvidia offers the models in various sizes (Nano, Super, and Ultra) as cloud-hosted microservices, making them accessible for enterprises of different scales. Major enterprise software players are already integrating Llama Nemotron models: Microsoft is incorporating them into its AI solutions, SAP is using them to enhance its AI copilot (Joule) for business applications, and ServiceNow is building them into its workflow automation agents. Consulting firms are on board too — Accenture added these models to its AI client toolkit, and Deloitte plans to use them in its “Zora” AI platform for enterprise decision support. For businesses, the takeaway is that state-of-the-art AI reasoning is becoming plug-and-play. Companies will soon be able to deploy AI agents capable of handling sophisticated tasks (from coding help to financial analysis) by leveraging these pre-trained reasoning models, instead of developing intelligence from scratch.
Tencent’s Upgraded T1 Model: In China’s fast-evolving AI scene, Tencent officially launched the latest version of its T1 language model on March 22, signaling intensifying competition among Chinese tech firms (Tencent launches T1 reasoning model amid growing AI competition in China | Reuters). Tencent’s T1 is positioned as a “reasoning model,” emphasizing improved logical coherence and very low hallucination rates (i.e., it seldom makes factual errors). The new T1 delivers faster responses and can better handle extended documents, according to the company. This launch comes on the heels of rival models from a company called DeepSeek that purportedly rival Western AI systems at a fraction of the cost. Tencent has been aggressively investing in AI; just a day before T1’s release, it announced plans to increase capital expenditure in 2025 to support more AI development. Notably, T1 is powered by Tencent’s Turbo S large language model unveiled late last month, which is optimized for speed. For businesses operating in or with China, Tencent’s rollout means more local options for AI services. Chinese enterprises can choose domestic models tuned to local language and needs, potentially at lower cost than importing U.S. models. This could spur faster AI adoption in sectors like finance and e-commerce in Asia—and Western firms should anticipate fiercer competition from Chinese companies armed with homegrown AI.
OpenAI’s Agent Tools and Connectors: OpenAI, the maker of ChatGPT, introduced a suite of new tools aimed at developers building AI agents and integrating AI into workflows. First, OpenAI launched a Responses API – a new interface that combines the best of its prior chat and assistant APIs into a simpler, more flexible format for multi-step tasks (OpenAI Launches New API, SDK, and Tools to Develop Custom Agents - InfoQ). With a single call to the Responses API, a developer can have an AI model use multiple tools and take multiple turns of conversation to solve a complex problem. Importantly, this API comes with built-in support for web browsing, local file search, and even operating a computer’s mouse and keyboard. This means an AI agent can automatically search the web for information, scan a company’s files, or perform actions on a PC as part of its reasoning – all under controlled settings. Alongside this, OpenAI released an Agents SDK (software development kit) to orchestrate these agentic workflows. The SDK helps define multiple agents that can hand off tasks to each other, and it includes safety guardrails (input/output checks) plus tools to visualize an agent’s decision steps for debugging. OpenAI signaled that such autonomous AI agents will become integral co-workers, handling complex, multi-step jobs in customer support, research, content generation, and more. Additionally, OpenAI is beta testing ChatGPT “Connectors” – integrations that allow business ChatGPT users to link the AI with internal apps like Slack and Google Drive. With Connectors, ChatGPT can answer questions using a company’s own documents or chat logs, staying within enterprise data silos. For example, an employee could ask ChatGPT to pull information from a project folder in Google Drive or summarize a Slack channel conversation, and the AI will securely retrieve that internal content. These developments from OpenAI indicate that enterprise AI is becoming deeply embedded: businesses will be able to custom-build AI agents that not only converse intelligently but also take actions across software systems. The caveat is ensuring robust governance—OpenAI’s inclusion of guardrails shows the need to manage risks when AI has such broad powers.
New AI Modalities – Speech and Multimodal: Tech firms also expanded AI capabilities beyond text. Google announced it is adding its Chirp 3 audio model to the Vertex AI cloud platform (UK must have global ambition in AI, DeepMind's Hassabis says | Reuters). Chirp 3 is a generative AI model for speech that produces voices with human-like intonation and expressiveness. In practical terms, businesses using Google’s Vertex AI will soon be able to generate high-quality, natural-sounding speech (for uses like virtual assistants, call center automation, or audiobook narration) without needing specialized in-house models. Meanwhile, Google’s Gemini AI (which powers its Bard assistant) received updates for image generation. According to Google developers, the latest Gemini 2.0 Flash model can create images natively as part of its responses (This week's AI industry updates: March 18, 2025), making Google the first major U.S. company to integrate text and image generation in one system. This multimodal capability means a user (or an app) could ask Gemini to “design a draft marketing poster for Product X” and get both the copy and a custom graphic in one go. Google also launched an open-source model named Gemma 3, touted as the most advanced AI that can run on a single GPU or TPU (a relatively lightweight deployment) while supporting 140 languages and some visual processing. For businesses, these developments in speech and multimodal AI open new possibilities: automated voice interfaces in multiple languages for customer service, on-the-fly content creation combining text and imagery for marketing, and cost-effective deployment of AI models on-premises or at the edge (since Gemma 3 can run on modest hardware). As these models become accessible, companies can leverage AI to generate rich content and interactions at scale, tailoring outputs to their brand voice and style.
3. Major Vendor Updates
Nvidia’s AI Hardware and Partnerships: Nvidia used its GTC 2025 conference to assert its dominance in AI hardware. CEO Jensen Huang revealed the next-generation Blackwell Ultra GPU – a powerhouse chip due in the second half of 2025, featuring larger memory to train and run even bigger AI models (Everything Nvidia announced at its annual developer conference GTC | Reuters). For enterprise buyers, this promises the ability to handle more complex AI workloads locally. Nvidia also previewed its future roadmap: new Vera Rubin AI chips and systems are slated for 2026 with radically faster chip-to-chip interconnects, improving performance in giant AI clusters. Looking further out, an architecture code-named Feynman is planned for 2028, underscoring the long-term commitment to push AI compute limits. Beyond chips, Nvidia announced DGX Personal AI Computers – essentially AI workstations for developers, built with Blackwell Ultra GPUs, to bring supercomputer-level AI capabilities to the desktop. Major PC makers (Dell, Lenovo, HP) will manufacture these, indicating businesses can soon equip AI developers with on-premises hardware that rivals cloud instances. Nvidia didn’t stop at computation: it introduced Spectrum-X and Quantum-X networking chips using silicon photonics to connect “AI factories” (large data centers) with millions of GPUs efficiently. For firms running their own data centers, this tech can significantly reduce energy costs and latency in AI training by speeding up data transfer between servers.
One partnership particularly noteworthy for infrastructure: Nvidia and Elon Musk’s new AI startup xAI joined a consortium with Microsoft, BlackRock, and others to invest in AI data centers and energy projects (Nvidia, Musk's xAI to join Microsoft, BlackRock and MGX to develop AI infrastructure | Reuters). The group, now called the AI Infrastructure Partnership, aims to deploy over $30 billion (potentially up to $100 billion) into scaling the backbone for AI applications. This includes funding cutting-edge semiconductor facilities and power solutions to support the surge in demand from AI like ChatGPT. For businesses, especially startups or smaller players, this consortium means the big firms are ensuring AI capacity will be available – likely easing fears of GPU shortages or cloud cost spikes. It also signals that AI development will increasingly require significant capital and infrastructure (with even energy companies like NextEra joining to help manage power needs). In practice, as this infrastructure comes online, companies of all sizes should benefit from more abundant and cheaper AI computing resources, either through cloud services or industry partnerships.
Oracle’s $5B Investment in UK Cloud for AI: Oracle, a major cloud vendor, announced plans to invest £5 billion (≈ $5B) in expanding its cloud infrastructure in the United Kingdom over the next five years (UK must have global ambition in AI, DeepMind's Hassabis says | Reuters). The company framed this as support for the UK government’s vision of AI innovation. Concretely, Oracle will build more data centers and cloud services capacity in Britain to meet growing demand from both public and private sector clients adopting AI. This move is significant for businesses in Europe: it reflects how cloud providers are positioning infrastructure regionally to address data residency and sovereignty concerns. For example, companies in regulated industries (like finance or healthcare in Switzerland) often require that sensitive data and AI workloads remain within certain jurisdictions. Oracle’s investment means more local options for hosting AI applications in Europe with guaranteed performance. It also underscores the global race among cloud vendors (AWS, Microsoft Azure, Google Cloud, Oracle, etc.) to capture AI workloads by building out capacity. Oracle’s expansion, alongside Google Cloud’s introduction of UK data residency for its new Google “Agentspace” productivity AI tool, suggests that major vendors are tailoring their offerings to comply with European privacy standards and to attract governments and enterprises that insist on local data control. Swiss businesses can expect similar offerings: more cloud zones in or near Switzerland and services that let them harness AI while keeping data under Swiss/EU jurisdiction.
Big Tech Expanding AI Ecosystems: OpenAI and Meta, two giants in AI, are looking beyond their home markets for growth. According to a report in The Information, both companies have been in talks with India’s Reliance Industries—a massive conglomerate — to collaborate on AI offerings (OpenAI, Meta in talks with Reliance for AI partnerships, The Information reports | Reuters). One discussed scenario is a partnership between Reliance’s telecom arm (Jio) and OpenAI to distribute ChatGPT in India, potentially pre-installing it on smartphones or integrating it with Jio’s services. OpenAI even floated the idea of lowering ChatGPT’s subscription price for certain markets to just a few dollars (from the standard $20/month) to broaden adoption. For Meta, which open-sourced its Llama models, Reliance could provide a pathway to enterprise customers and the massive Indian user base. Additionally, Reliance is considering hosting these AI models in its own upcoming data center (a 3GW facility in Jamnagar) to keep Indian user data local. This highlights a key business point: local partnerships and pricing strategies will shape AI global expansion. For Swiss companies (especially multinationals or those targeting emerging markets), the lesson is to consider localized AI deployment. Just as OpenAI might customize pricing and infrastructure for India, Swiss firms should think about how to tailor AI-driven products for different regions, conforming to local regulations and cost sensitivities.
4. AI Governance and Regulation
Calls for International Standards: As AI systems become ubiquitous, questions around data usage and intellectual property are front and center. Demis Hassabis, co-founder of Google DeepMind, urged the creation of international standards for using copyrighted material in AI training (UK must have global ambition in AI, DeepMind's Hassabis says | Reuters). Speaking in London, Hassabis noted that AI models are global by nature — they ingest data from everywhere and are used everywhere—so a patchwork of national rules won’t suffice. His concern addresses a scenario many businesses worry about: AI models trained on copyrighted text or images without permission. Creative industries fear loss of control and revenue if AI can churn out content “in the style of” living artists or authors. Hassabis’s call echoes these concerns and suggests that major AI developers would welcome clear, consistent rules on what data is fair game for training. For example, a global standard might require AI firms to document and disclose training datasets or to obtain licenses for certain content. For businesses, such standards would reduce legal uncertainty when deploying AI—knowing that the models they use comply with agreed norms on data usage. It could also level the playing field, preventing less regulated jurisdictions from gaining an unfair advantage in AI development by using data in ways not allowed elsewhere.
Transatlantic Cooperation on AI: Government leaders are increasingly viewing AI through a geopolitical lens. The UK’s Prime Minister, Keir Starmer, said in a recent U.S. visit that the United States and Britain are working on an economic agreement focused on advanced technologies, including AI (UK must have global ambition in AI, DeepMind's Hassabis says | Reuters). While details are scant, this indicates a desire among Western allies to align on AI innovation and possibly regulation. For example, such a deal might involve joint research programs, harmonized standards (so AI systems approved in one country can be easily approved in the other), or coordinated security measures to control sensitive AI exports. For companies operating in both the US and Europe, this is a positive sign—regulatory alignment would ease compliance burdens. We might expect fewer conflicting rules and more mutual recognition in areas like AI safety certifications or data protection frameworks for AI. Additionally, a coordinated stance could help Western firms compete with the massive government-backed AI initiatives in China by pooling talent and resources across the Atlantic.
Cautious Approach vs. Innovation Incentives: Different countries are striking different balances between regulating AI risks and fostering innovation. This was evident in a Swiss government communication that came to light this week. Switzerland’s State Secretariat for Economic Affairs (SECO) reassured the U.S. in a comment that Switzerland has no onerous AI-specific regulations or digital taxes that would burden tech companies (Schweiz beschwichtigt USA mit fehlender Regulierung für KI und Plattformen - datenrecht.ch – das Datenrechts-Team von Walder Wyss). In fact, Switzerland positions itself as a bridgehead for U.S. tech firms into Europe, highlighting its business-friendly environment with minimal additional rules for AI and online platforms. The subtext is clear: while the EU debates strict AI rules, Switzerland is signaling a lighter regulatory touch to attract investment and avoid trade conflicts. This laissez-faire stance can benefit businesses by allowing more freedom to experiment with AI. A company operating in Zurich might face fewer constraints on deploying AI solutions (for instance, no local equivalent of the EU’s high-risk AI system requirements—yet). However, it’s a double-edged sword; as AI alliance groups point out, a lack of regulation can also mean less protection for incumbents in creative industries or consumers (we will see how Swiss lawmakers are addressing that in the Swiss section below). Globally, we see a spectrum: the EU’s AI Act (which entered into force in 2024) takes a precautionary approach with strict rules for high-risk AI, while countries like the UK and Switzerland lean toward innovation-first policies for now. Businesses need to stay agile, adapting their compliance strategies country by country.
Industry Self-Governance and AI Safety: In tandem with government action, the AI industry itself is moving toward self-regulation through best practices. OpenAI’s new Agents SDK, for example, includes built-in safety checks to prevent “irrelevant, harmful, or undesirable behavior” when AI agents use tools or produce outputs (OpenAI Launches New API, SDK, and Tools to Develop Custom Agents - InfoQ). Developers can define guardrails so that an AI agent doesn’t, say, execute a risky command or expose confidential data. Many AI vendors are similarly emphasizing “responsible AI” features—Microsoft’s Azure OpenAI service, Google’s Vertex AI, etc., all tout monitoring and control options. This is partly to preempt stricter laws by showing that the industry can police itself. Another development is the work on AI auditing and evaluation: research this week in Nature discussed metrics for how well AI systems handle long-term tasks, reflecting growing academic focus on AI benchmarking for safety and reliability. For businesses implementing AI, it’s wise to adopt these emerging best practices early. Not only does it reduce the risk of something going wrong (a rogue AI action or a PR fiasco from biased outputs), but it also prepares the company for eventual regulations that might mandate such controls. Furthermore, demonstrating strong AI governance can be a market differentiator, especially in fields like finance or healthcare where trust is paramount.
In summary, the governance landscape in late March 2025 is one of proactive proposals but pending action. Leaders like Hassabis are voicing the right issues (IP rights, global cooperation), and some governments are aligning on tech strategies, yet concrete regulations (outside of the EU’s AI Act) are still in flux. Businesses should engage with policymakers via industry groups to ensure their interests (like needing clarity on data usage) are represented, and concurrently invest in internal AI oversight to be ready for the likely wave of regulations in the next 1–2 years.
5. Breakthrough Research and Innovations
AI “Co-Pilots” for Science: Beyond commercial product launches, this week saw AI breaking new ground in scientific research. Google DeepMind showcased progress on an AI research assistant that can generate novel scientific hypotheses (Accelerating scientific breakthroughs with an AI co-scientist). This multi-agent system, built on the Gemini 2.0 model, acts as a “virtual scientist” – it can propose potential experiments or solutions that human researchers might overlook. For example, given a trove of data, the AI might suggest a new therapeutic target for a disease or a unique material for carbon capture, by drawing connections across disciplines. While still experimental, such tools hint at a future where R&D teams in pharmaceuticals, chemistry, or engineering routinely use AI to accelerate innovation. Early evidence of AI’s scientific prowess emerged when an AI system dubbed AI Scientist successfully generated a peer-reviewed scientific paper with minimal human input. For businesses in the biotech and industrial sectors, integrating AI at the lab stage could dramatically shorten discovery cycles and cut costs—potentially leading to faster time-to-market for new drugs or materials. It also means that companies will need employees capable of collaborating with AI on research, interpreting AI-generated ideas, and validating them. Those who master this synergy will likely outpace competitors.
Genomic AI – Evo 2 Model: In an exciting breakthrough at the intersection of AI and biology, researchers from the Arc Institute, Stanford University, and NVIDIA announced Evo 2, the largest AI model for genomic data to date (Biggest-ever AI biology model writes DNA on demand - Nature) (NVIDIA & Arc Institute launch AI model to predict DNA, RNA, and protein structures.). Evo 2 was trained on the DNA of over 128,000 species — encompassing nine trillion genetic bases—and can both predict and design genetic sequences across all life domains. Remarkably, Evo 2 is fully open-source, with its model parameters and training code available to scientists worldwide. What can it do? For one, it can predict the effect of genetic mutations, which is huge for healthcare: researchers could identify which DNA changes cause disease or conversely engineer beneficial traits. It can also assist in designing synthetic genes and proteins, potentially revolutionizing biotech by enabling AI-guided creation of new enzymes, biofuels, or therapeutics. NVIDIA heralded this as a leap in generative genomics, noting it will help tackle problems in healthcare and environmental science that were previously unimaginable. Evo 2 can handle sequences up to 1 million DNA letters long in one go, which allows analysis of complex genomic regions and interactions. For context, a human genome is about 3 billion letters; analyzing chunks of that at million-scale tokens is very powerful for understanding gene regulation and cellular mechanisms. The business implications are vast: pharmaceutical companies could use Evo 2 (or its derivatives) to speed up drug target discovery or even personalize medicine to a patient’s genome. Agricultural firms might develop climate-resilient crops by having AI suggest genetic tweaks. While Evo 2 itself is a research model, it exemplifies how AI is accelerating life sciences, a domain highly relevant to Switzerland’s strong pharma and biotech industry.
AI Performance and Benchmarks: Finally, a conceptual innovation worth noting is in how we measure AI’s progress. A report in Nature highlighted a new metric for evaluating AI systems on lengthy, complex tasks (AI could soon tackle projects that take humans weeks - Nature). Unlike traditional benchmarks that focus on single-turn Q&A or short tasks, this metric assesses an AI’s ability to plan and execute multi-step projects that could take humans weeks. This is timely, as we now have AI “agents” that can schedule a week’s worth of actions or manage an ongoing project (e.g., an AI system that autonomously runs a simulation experiment over days). Early results show AI improving rapidly on such metrics, though some researchers urge caution in extrapolating too far into the future. Why does this matter for businesses? It points to the emergence of AI project managers or strategists. In the not-so-distant future, companies might assign certain routine projects entirely to an AI agent — confident that success isn’t just a roll of the dice but can be quantitatively predicted because the AI scored well on these new benchmarks. It’s part of the professionalization of AI: we’re setting standards and performance indicators for AI as we would for human employees. Businesses should stay informed about these advancements; understanding AI’s capabilities through standardized metrics will help in deciding which business processes to automate or hand over to AI, and which still require the human touch.
6. Swiss-Specific Developments
Federal Administration’s AI Strategy: On March 21, the Swiss Federal Council was briefed on a new strategy for the use of AI in the federal administration (Bundesverwaltung legt Grundsätze für Einsatz von KI in der Verwaltung fest). The Federal Chancellery has been tasked with formulating an implementation plan by the end of the year. The strategy lays out three guiding principles for AI in government: building competencies, ensuring trustworthy use, and improving efficiency. In practice, this means training civil servants in AI skills, using AI systems in a legally and ethically compliant way, and deploying AI to streamline bureaucratic processes. For example, routine paperwork or data analysis in federal offices could soon be handled by AI, freeing officials for higher-value work. The aim is also cost savings—AI should help save time and money in delivering public services. For businesses, this is encouraging: a tech-savvy government can cut red tape and potentially offer new digital services (like faster permit approvals or AI-powered information portals for companies). It also signals opportunities for the private sector to partner on govtech solutions, as Bern will likely rely on Swiss AI vendors and consultants to implement these measures. Importantly, the commitment to trustworthy AI means there will be an emphasis on transparency and legal compliance, so companies providing AI to the government should align with those values (e.g., ensuring algorithms are non-discriminatory and data is handled according to privacy laws).
IP Protection in the Age of AI: Switzerland is moving to protect its creative industries from unlicensed AI use of content. In a notable decision on March 20, the Council of States (Ständerat) unanimously approved a motion titled “Better protection of intellectual property from AI misuse” (News - SONART - Musikschaffende Schweiz). This motion (put forward by Councillor Doris Fiala Gössi) calls for requiring that AI developers or users obtain permission from rights holders if they use copyrighted works to train AI models or generate content. The country’s creative sector alliance, KIK, applauded this as a “strong signal” in defense of artists, writers, and media producers. They argue that current AI practices—scraping books, songs, or images without licensing—threaten creators’ incomes and reputations. The Federal Council had acknowledged the issue in a February report but was hesitant about concrete steps, tentatively mentioning any regulations might only come by 2029. The Ständerat’s decisive vote speeds up the timeline and puts pressure on the National Council (Nationalrat) and the Federal Council to act much sooner. For businesses, especially those in media, publishing, and design in Switzerland, this move, if it becomes law, would provide clearer legal grounds to control how AI uses their content. It may result in a framework where companies can license their data or creative works to AI firms for a fee, opening a new revenue stream. On the flip side, Swiss firms developing AI will need to build compliance into their model training—potentially curating datasets to exclude unlicensed Swiss copyrighted content or implementing filters to avoid IP infringement when generating outputs. Overall, Switzerland appears to be carving a path that balances innovation with creators’ rights, and companies should prepare for stricter enforcement of IP rules in AI.
AI Adoption Among Swiss Consumers: A new survey by Comparis, released March 18, highlights how rapidly AI chatbots have been embraced by the Swiss public (Immer mehr Schweizer nutzen KI-Chatbots | Netzwoche). Over 62% of Swiss respondents said they have used AI chatbots like ChatGPT or Google’s Gemini at least once, up from about 50% a year prior. The growth is especially pronounced among young adults: an impressive 81% of 18–35 year-olds in Switzerland have tried these AI tools. Even among the 36–55 age group, two-thirds have used them, and a notable one-third of those over 56 have dabbled with AI assistants. This broad usage suggests that Swiss consumers are increasingly comfortable turning to AI for information searches, which was cited as the most common use case in the survey. However, the survey also found hesitancy in using AI for sensitive matters—Swiss people are “wenig beliebt” (not keen) on using chatbots for private or health-related data, indicating trust concerns in those domains.
For Swiss businesses, these findings carry several implications. First, the high adoption rate means AI literacy is growing among customers. Companies can consider integrating AI chat interfaces on their websites or apps, knowing that many users will find it natural to interact in that way. In fact, offering an AI chat assistant could become a competitive differentiator in customer service, as users begin to expect instant, intelligent responses 24/7. Second, the caution around personal data means trust is paramount: firms deploying AI (especially in finance or healthcare) should be transparent about data use and security. It might be wise to clarify, for instance, if an insurance chatbot is not storing personal details or if a medical AI is validated by professionals. Lastly, the near-ubiquity of AI usage in Switzerland’s younger generation signals a future workforce comfortable with AI tools. Swiss companies can leverage this by incorporating AI in internal workflows (like using GPT-style assistants for drafting reports or coding) to boost productivity, as many employees will already be familiar with the interface and capabilities. The trend also encourages businesses to invest in training programs for staff on how to effectively use AI tools – maximizing benefit while understanding limitations.
Maintaining Switzerland’s AI Edge: The contrast between Switzerland’s light regulatory stance and its moves to address specific issues like IP reflects a strategic effort to foster AI progress while protecting core values. Swiss universities (ETH Zurich, EPFL) and startups are active in AI research, and the country aims to remain a hub for innovation. The federal administration’s principles for trustworthy AI use, combined with parliament’s actions on copyright, indicate that Switzerland wants “human-centric” AI that respects rights and societal values. For example, Swiss authorities might soon publish guidelines on AI transparency or encourage the development of auditing tools for algorithms – creating a market for compliance tech. Businesses here should stay engaged with these national discussions. This week’s developments show that the government is receptive to input (the IP motion came from industry lobbying via KIK). By contributing to public consultations or industry alliances, companies can help shape pragmatic AI policies that both spur innovation and build public trust. In a country known for quality and precision, a Swiss brand of AI governance could become a selling point – imagine being able to tell your customers that your AI-powered service follows “Swiss-certified ethical AI standards.” Given Swiss consumers’ growing usage but cautious trust, aligning with such standards might soon be essential for market acceptance.
7. Conclusion
In conclusion, the AI developments of this week highlight a dual imperative for businesses: innovation and responsibility. Those that innovate by integrating the latest AI capabilities will unlock growth and efficiency – whether through smarter automation, better customer insights, or new AI-powered offerings. At the same time, doing so responsibly, with an eye on compliance, ethical use, and alignment with evolving regulations, will be crucial to sustain that growth. Switzerland’s business community, with its tradition of quality and trust, is well-positioned to lead in this new era by deploying AI that is both cutting-edge and conscientious. The events of this week make it clear that AI is not a distant future concept; it’s here now, transforming business in real time. Companies that recognize its strategic value – and act on it – will thrive in the years ahead.