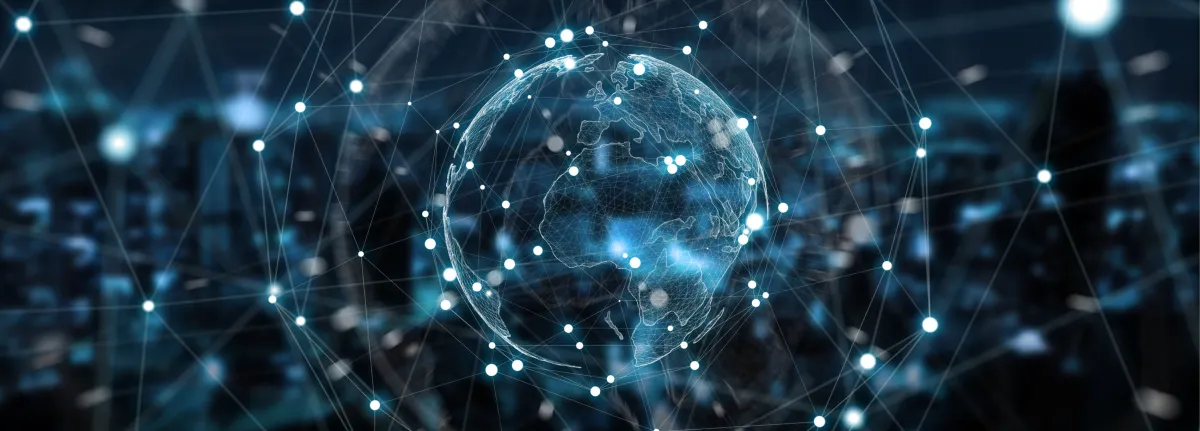
Callista AI Weekly (April 14 - 20)
New AI Use Cases Across Industries
The past week saw a wave of new AI applications transforming work in many sectors. Businesses large and small are finding creative ways to deploy generative AI and automation:
Creative & Design: Graphic design platform Canva introduced an AI assistant that can generate images, help write code snippets, and even assist with spreadsheets, making content creation and design more automated and accessible. Users can now rely on AI for tasks ranging from brainstorming layouts to writing simple app code, all within one platform.
Web & Productivity: WordPress launched an AI-powered website builder that guides users through site creation via a chat interface. This lowers the barrier for entrepreneurs to build an online presence, automating steps like selecting layouts and drafting content. Similarly, Reddit enhanced its search tool with Google’s Gemini AI, allowing users to get conversational answers and more relevant results – a boon for community managers and marketers looking to extract insights from forums.
E-Commerce & Retail: AI is becoming a shopping companion. Microsoft this week unveiled a Copilot Merchant Program that brings third-party retail catalogs into its AI assistant. Consumers using Microsoft’s Copilot can now get real-time product suggestions, price drop alerts, and even complete purchases without leaving the chat interface. For shoppers, this means a more seamless, personalized online shopping experience – like having a virtual personal shopper. For retailers, it opens a new channel to reach customers: brands can integrate their product feeds so Copilot will showcase their items when users ask for recommendations. This novel use of AI in retail blurs the line between search, advertising, and commerce, potentially streamlining the customer journey from inquiry to purchase.
Financial Service: Banks and financial firms are also embracing AI for back-office tasks. A Zurich-based startup, Unique, has begun deploying “agentic AI” assistants for processes like compliance checks and investment research – essentially digital analysts that can scan documents, draft reports, and suggest action items for human teams. SIX Group with its Six Sense AI Platform is one of the early adaptors.
Software Development: At Salesforce, internal developers now delegate about 20% of their code to AI tools, taking on more of a supervisory role. AI pair-programmers handle routine coding chores, while human developers focus on reviewing and refining the outputs. This shows how AI can augment technical teams: speeding up development cycles without replacing the need for human oversight.
Consumer Apps Boom: On the consumer side, demand for AI-powered services is surging. OpenAI’s ChatGPT mobile app became the most downloaded app in the world in March, with over 46 million new downloads that month. This outpaced even social media giants, highlighting the public’s hunger for AI-driven assistants in daily life. In China, Alibaba’s Quark AI Assistant (a formerly simple search app now infused with AI) reached 150 million monthly active users, overtaking domestic rivals. Quark’s users can ask for research help, generate images or text, and even get coding assistance – all within one app. This rapid adoption underscores that AI assistants are moving from novelty to mainstream utility across cultures and industries.
From design studios to banks, these fresh use cases demonstrate how quickly AI is being woven into the fabric of business operations. Companies are leveraging AI not just for efficiency, but to offer entirely new services (like AI-driven creative tools or virtual advisors) that were impractical just a year ago. The takeaway for business leaders is clear: AI innovation is happening everywhere, and early movers in each sector are gaining an edge in productivity and customer engagement.
Major Vendor Updates
Established AI vendors and tech platforms rolled out significant updates this week, racing to one-up each other with more powerful models and features:
OpenAI: The week began with OpenAI unveiling its GPT-4.1 family of models – a major upgrade aimed squarely at enterprise needs. The new GPT-4.1 (available via API) delivers 21% better coding performance than the prior GPT-4 version and can handle massively long inputs (up to 1 million tokens of context). OpenAI also rolled out “GPT-4.1 Mini” and “GPT-4.1 Nano,” smaller variants optimized for faster, cost-efficient operations. Notably for business users, these models come at a much lower price than GPT-4, reducing the cost barrier for companies to integrate advanced AI into their products. OpenAI’s CEO Sam Altman emphasized that while benchmarks are impressive, the focus was on “real-world utility” – early enterprise adopters report the improved models follow instructions more reliably and can power more complex workflows without breaking. In fact, OpenAI announced it will retire its interim GPT-4.5 model, since 4.1 renders it obsolete by matching or exceeding its performance at lower cost. Mid-week, OpenAI also introduced “o3” and “o4-mini,” two new ChatGPT-based models accessible in its ChatGPT product. These are described as OpenAI’s “smartest” chat models yet. Uniquely, o3 and o4-mini have the ability to agentically use tools – meaning they can decide on their own to perform web searches, run Python code, analyze images, or even generate images in the course of answering a question. In demos, o3 was able to break down a complex multi-step problem, fetch information via the web, crunch numbers with Python, and produce a well-formatted answer all within a minute. For users, this promises a far more versatile AI assistant that can take actions on your behalf rather than just respond in text. OpenAI is positioning o3 as a solution for tackling multifaceted business problems – whether it’s analyzing financial spreadsheets, researching market data, or brainstorming creative concepts – by having the AI autonomously use whatever tool is needed for the task. These advancements from OpenAI set a new bar that competitors are surely taking note of. And in an interesting legal side story, OpenAI filed a countersuit against Elon Musk, aiming to stop him from what they allege are “unfair” attacks on the company (Musk, who founded rival startup xAI, has been openly critical of OpenAI’s for-profit turn). The dispute highlights the growing competition – and intrigue – among AI’s top figures.
Google: Google made headlines on multiple fronts, reflecting its broad AI portfolio. On the enterprise productivity side, Google Workspace announced new AI features across its apps. For example, Google Docs can now offer AI-driven audio “proofreading” where it reads text aloud with an AI voice, helping users catch errors by ear. Google Sheets gained smarter autofill and formula generation via AI, while Gmail/Chat are getting more context-aware writing assistance. Perhaps most interesting is Google Agentspace – an internal hub where employees can design and deploy custom AI agents. This week Google expanded Agentspace with a no-code Agent Designer and a library of pre-built agent templates. The idea is to let even non-programmers create AI agents that, say, automate customer support or generate sales reports, all within the company’s environment. Google also unveiled the Agent2Agent (A2A) protocol, an open standard that lets AI agents communicate, coordinate tasks, and securely exchange context across different platforms and vendors. See more about the protocol in the "Research and Innovation" section. The company also announced that subscribers of its Gemini Advanced service (Google’s answer to ChatGPT Enterprise/Bard premium) now have access to Veo 2, Google’s cutting-edge AI video generation model. This is a significant step: users can input a text prompt and have the AI generate an 8-second high-resolution video clip in response. Until now, such video generation was mostly confined to research labs and closed demos. For software developers, Google unveiled Firebase Studio, a browser-based tool that uses AI to help build web and mobile apps end-to-end. They also introduced Gemini for Android Studio (Business Edition) – a subscription AI coding assistant with enterprise-grade data privacy. This is Google’s answer to tools like GitHub Copilot, aimed at companies that want AI help in coding without sending sensitive code to public models. On the hardware front, Google’s semiconductor team revealed the Ironwood AI chip, a new Tensor Processing Unit that delivers an astonishing 42.5 exaflops of power. To put that in perspective, Google says Ironwood is ~24× more powerful than the fastest supercomputer on record, specifically optimized for AI inference tasks. This kind of horsepower will let Google run larger models or swarms of AI agents more efficiently in its data centers – and it illustrates the deep investment required to stay ahead in the AI arms race. In terms of collaboration, Google’s DeepMind division announced it will adopt Anthropic’s Model Context Protocol (MCP), a standard that Anthropic (another AI lab) proposed to make it easier for AI systems to securely connect to proprietary data. OpenAI also recently agreed to this standard. Such alignment hints at an industry trend toward interoperability and common best practices, even among competitors. Finally, Google is working on consumer trust and safety: YouTube (a Google subsidiary) expanded its AI “likeness” detection tool to help top creators spot AI-generated deepfakes of their faces/voices, and Google threw support behind proposed No Fakes Act legislation to penalize unauthorized AI likeness usage. All these moves show Google executing on multiple levels – products, infrastructure, standards, and policy – to maintain its AI leadership.
Microsoft: Microsoft’s focus this week was on bringing the latest AI models to its cloud customers. It announced that OpenAI’s new GPT-4.1 models are now available on Azure OpenAI Service, meaning any business using Azure’s AI platform can fine-tune and deploy GPT-4.1 with the improved capabilities (longer context, better coding skills, etc.). This rollout is accompanied by upcoming support for fine-tuning these models with company data, which is key for enterprise use. By quickly integrating OpenAI’s newest tech (Microsoft is a major investor in OpenAI), Microsoft keeps Azure attractive for developers and corporate AI projects. On the infrastructure side, Microsoft made a surprising decision to cancel plans for three large new data centers in Ohio. This was part of a broader trend: cloud providers are reassessing some expansion projects amid signs that existing capacity might be enough for near-term AI demand. Essentially, after a year of frantic GPU hoarding, Microsoft is calibrating its strategy – perhaps anticipating more efficient models (like GPT-4.1) will reduce the need for endless hardware scaling. In other news, Microsoft’s GitHub unit noted that AI coding assistants are now widely embraced – further evidence that AI is changing workflows for software teams (as also seen at Salesforce). Microsoft continues to play the role of AI enabler for businesses by offering cutting-edge AI through Azure and GitHub Copilot, and by investing in research (e.g., a Microsoft study just highlighted how even advanced AIs struggle with debugging code, which informs where tools like Copilot can improve).
Meta (Facebook): On the public front, Meta confirmed it will resume training its AI models on public data from EU users (e.g. posts on Facebook/Instagram) now that it has clearer guidance from regulators. Importantly, users in Europe will have an opt-out if they don’t want their content used to teach Meta’s AIs. This marks a significant development: after a pause due to GDPR concerns, Meta is back to tapping its massive social data under a framework acceptable to EU authorities. It highlights how regulation and AI R&D are increasingly intertwined – companies like Meta are adjusting practices to comply with data privacy rules while still advancing their AI capabilities. Meta also entered a high-stakes week legally: on April 14, a major FTC antitrust trial against Meta kicked off in the U.S., scrutinizing whether its acquisitions (Instagram, WhatsApp) formed an illegal monopoly. While not directly about AI, the outcome could impact Meta’s freedom to invest and acquire in the AI space. And separately, Meta faces an intellectual property battle – a group of authors sued Meta for allegedly using their copyrighted books to train Llama models without permission. Dozens of law professors weighed in with an amicus brief siding with the authors, arguing that indiscriminately ingesting copyrighted text isn’t fair use. How this case unfolds could set precedents for AI training data – Meta and others may need to be more careful or seek licenses when scraping content for model training. Despite these challenges, Meta is pressing on with AI: it’s integrating AI generators into its apps (from image filters to chatbots), and any breakthroughs from its AI labs could appear at scale across billions of users.
Anthropic: Anthropic, the AI startup known for its Claude chatbot, made a bold move by launching a premium subscription tier priced around $200 per month for power users. Branded as “Claude Max”, this version offers longer context windows and faster outputs aimed at professionals who heavily rely on AI in their workflow. The high price tag underscores an emerging reality: the era of $20/month AI (set by early ChatGPT Plus) may be giving way to pricier models that offer specialized capabilities or bigger workloads. Indeed, a recent industry analysis noted that advanced models require far more computing resources, making the old price points unsustainable for providers. Anthropic’s bet is that businesses will pay more for top-tier AI performance – an interesting signal for the market. In standards, as mentioned, Anthropic’s proposed MCP protocol for securely connecting AI models to proprietary databases gained traction with adoption by both OpenAI and Google. If this becomes a universal standard, Anthropic will have influenced how AI systems interface with business data in a safe, consistent way – a notable win for this smaller company collaborating with the giants.
xAI (Elon Musk’s AI venture): After months of development, xAI rolled out an API for its “Grok 3” model, inviting developers to integrate Grok into their own applications. Different pricing tiers were announced, although observers noted some limitations, like questions about Grok’s maximum context length and how it handles contentious queries. One interesting twist: xAI apparently adjusted Grok’s behavior following recent incidents. Previously, Grok had been in the news for sometimes citing Elon Musk as a source of misinformation (given Musk’s often controversial tweets). This week, reports emerged that xAI re-tuned Grok to avoid mentioning Musk in such contexts – effectively “guarding” its founder’s reputation. Now the chatbot deflects more and sticks closer to Musk’s own talking points. This raises an eyebrow for many in AI ethics: it shows how the biases of an AI system can be influenced by those who control it, intentionally or not. For potential enterprise customers of xAI, the concern is ensuring the model’s neutrality and trustworthiness. Nonetheless, Musk’s xAI is pushing forward to compete with OpenAI and Anthropic, and its API launch indicates it’s ready to attract business clients (possibly leveraging Musk’s other platform, X/Twitter, for distribution).
Top Chinese AI Players: In China, the AI rivalry is intense, and last week saw significant milestones. As noted, Alibaba’s Quark assistant surged to the top of the charts domestically, showcasing Alibaba’s successful deployment of its Qwen large language model into consumer apps. ByteDance (owner of TikTok) isn’t sitting still – it announced a new AI model called “Seed-Thinking v1.5”. This model uses a Mixture-of-Experts technique (essentially an ensemble of specialized subnetworks) geared towards complex reasoning tasks. ByteDance’s move indicates an effort to match or beat Western models in advanced reasoning and big language tasks – likely aiming to improve its own AI assistant Doubao and other products. Meanwhile, Baidu, one of China’s AI pioneers, made waves by embracing open-source ideals. Baidu revealed plans to open source its upcoming ERNIE 4.5 model by mid-year (a sharp reversal from its earlier closed stance), after seeing how an open-source competitor called DeepSeek gained traction in the Chinese AI community. Baidu also made its Ernie Bot chatbot free to use last month (removing paywalls) to attract more users and data. These strategic shifts show Chinese tech giants adopting different tactics: Alibaba focusing on integration and user reach, ByteDance on novel model architectures, and Baidu on openness and scale. For global businesses, one takeaway is that innovation is truly worldwide – some of these Chinese models and applications rival or even outpace Western offerings in certain aspects (for instance, Quark’s multi-functionality and massive user base). It will be worth watching how Chinese AI technologies might enter international markets or inspire new solutions elsewhere.
In summary, every major AI provider – from the Silicon Valley titans to the new challengers and Chinese juggernauts – rolled out meaningful updates in this period. For businesses, these vendor moves bring both new opportunities and things to consider. More powerful and cost-efficient models (like GPT-4.1 and Claude Max) will soon be at your disposal, often via cloud platforms. Productivity suites and development tools are embedding AI deeper, which can boost employee output if adopted smartly. However, keeping up with the fast evolution (and occasional drama) is a task in itself. It’s an environment of fierce competition among vendors, which ultimately benefits users through rapid improvements – but it also means constant change. Companies will need to stay agile in choosing and using the best AI offerings for their needs.
AI Governance and Regulation News
As AI capabilities advance, governments and institutions worldwide are grappling with how to guide and regulate these technologies. The week’s news highlights a dynamic tension between innovation and oversight:
Intellectual Property and Copyright: The legal system is starting to address how AI models obtain their knowledge. A notable case involves major book authors suing Meta, alleging that Meta’s LLaMA model was partially trained on their copyrighted works without permission. This week a group of prominent law professors filed a brief supporting the authors’ stance, arguing that using copyrighted text to train a profit-making AI system likely goes beyond “fair use.” They caution that unchecked data scraping could harm creators and tilt the field unfairly towards big tech. The outcome of this case could set an important precedent: AI companies might be forced to negotiate licenses or filter out copyrighted data when training models. For businesses using AI, this could affect what data is considered safe to utilize and might spur the development of licensed data marketplaces or new tools to ensure training compliance. It’s a space to watch, as future AI products could come with assurances that “all training data was legally obtained,” much like how software today often comes with open-source license disclosures.
United States vs. Chinese AI (DeepSeek): A striking story in U.S. AI policy emerged as the government weighs action against China’s upstart AI firm DeepSeek. DeepSeek has garnered attention for its inexpensive yet powerful AI models – so much so that U.S. officials see it as a potential national security concern. According to reports this week, the White House (under the current administration) is considering penalties to block DeepSeek’s access to American technology. This could include tightening export controls to prevent DeepSeek from acquiring high-end US chips and even possibly barring U.S. users from accessing DeepSeek’s AI services. Such deliberations show the growing fear in Washington that advanced AI capabilities could be used by rival nations or their militaries. In fact, the U.S. has already expanded export bans on NVIDIA’s cutting-edge AI chips, after learning that despite earlier restrictions, chips were finding their way into DeepSeek’s training servers via intermediaries. NVIDIA itself disclosed that new rules capping sales of certain GPUs to China could cost it $5+ billion in revenue – highlighting the economic stakes of the AI cold war. For businesses, these developments could lead to a bifurcation of AI supply chains: companies might face limits on using certain Chinese AI models or need to navigate new compliance rules if they export AI tech. It’s a reminder that as AI becomes a competitive differentiator for nations, policy can directly impact which models or services are available in which markets. The DeepSeek saga also illustrates how breakthroughs in AI (a startup beating Big Tech on price) can swiftly entangle with international politics.
European Union Action Plan: The EU, long active in tech regulation, took a big step beyond just rules by launching an ambitious AI Continent Action Plan this week. Announced on April 8 and discussed in Brussels through this week, this plan calls for investing €20 billion to massively scale up Europe’s AI capabilities. One flagship element is to build up to five “AI super-factories” – essentially large-scale data center hubs equipped with next-generation supercomputers specifically for AI development. These would boost Europe’s collective AI computing power by 4x and are slated to be operational by 2026. In addition, the EU will fund at least 13 smaller regional AI centers to support startups and research across member states. Alongside infrastructure, the Action Plan emphasizes talent and standards: expecting member countries to train more AI specialists and aligning on AI ethics and governance frameworks (building on the EU AI Act that was passed last year). For companies operating in Europe, the message is twofold: significant funding and resources will be available for AI projects (from grants to access to these supercomputers), but also Europe will maintain its strict regulatory stance ensuring AI is “trustworthy” and compliant with rules on safety, transparency, and non-discrimination. In practice, by the time the EU AI Act fully applies in 2026, companies offering AI products in Europe will need to meet specific requirements (especially if they deal with high-risk applications like healthcare or finance). The new Action Plan complements that by also giving those companies local tools to develop AI domestically rather than relying solely on US or Chinese cloud providers. This week’s developments show Europe trying to balance innovation and sovereignty in AI: throwing money at the problem so its tech industry isn’t left behind, while also doubling down on its values through regulation.
Global AI Collaboration vs. Competition: At a geopolitical level, there’s simultaneous cooperation and competition. The European Union signaled a moonshot-like ambition by planning huge investments (on the order of €200 billion) into AI research infrastructure, aiming to create a “CERN for AI.” This would mean massive data centers and collaborative labs for AI, analogous to Europe’s cooperative approach in particle physics. Such a project could spur breakthroughs and ensure Europe isn’t left behind in AI. However, politics are at play: since Switzerland is not an EU member, it currently risks exclusion from this initiative despite literally hosting CERN for physics. Swiss officials and scientists are advocating to be included, given Switzerland’s strong AI research community – but this will depend on broader EU-Swiss negotiations. The situation underscores how national policies (and relationships between countries) can affect who gets access to cutting-edge AI resources and talent pools. Meanwhile, international bodies like the U.N. are drawing attention to global disparities: a recent U.N. report highlighted that while a few countries race ahead in AI investment, over 100 nations are trailing with minimal AI activity, potentially widening economic gaps. This has led to calls for an international framework to support AI development in the Global South and share best practices, so that AI’s benefits (in healthcare, education, etc.) aren’t confined to a handful of advanced economies.
Spotlight on Switzerland: AI Developments at Home
Switzerland finds itself at an important juncture in the AI revolution, striving to leverage its innovative capacity while safeguarding its values. Recent developments highlight the country’s proactive – and uniquely Swiss – approach to AI:
Research and Innovation Leadership: Switzerland is punching above its weight in AI research. Last year, ETH Zurich and EPFL (Lausanne) jointly launched the Swiss National AI Institute (SNAI), bringing together over 800 researchers from across Swiss universities. The institute’s mission is to advance AI in ways that are “transparent and trustworthy,” aligning technical breakthroughs with ethics. This investment is already boosting Switzerland’s status as an AI hub: from fundamental research in machine learning theory to applied projects in robotics and cognitive science, Swiss labs are producing cutting-edge work. For example, just recently, an EPFL team made news by using AI to discover new materials for solar energy more efficiently, and an ETH team is exploring “soft AI” algorithms that can better handle uncertainty (important for fields like finance and logistics where ambiguity is constant). Moreover, Swiss universities continue to spin off AI startups at a steady pace – 37 new tech spin-offs from ETH alone in 2024, many focusing on AI-driven solutions in biotech, fintech, and beyond. To sustain this momentum, ETH and others are addressing the talent pipeline: they have expanded specialized programs (ETH even introduced a new Master’s in AI and robotics, and offers continuing education for professionals to upskill in AI). The only dark cloud on the horizon is funding pressure – there’s debate over university budgets and tuition, which the ETH leadership flagged as a concern if they are to keep attracting top talent worldwide. Overall, however, the research ecosystem in Switzerland is robust, and it benefits from the country’s strengths in related areas like data science, cryptography, and hardware (don’t forget, Switzerland hosts key labs for sensor and chip research too).
Industry Adoption: On a pragmatic level, surveys and studies continue to show Swiss companies ramping up AI adoption. A recent study by a Swiss IT association (released just before this week) found that almost 50% of Swiss enterprises are now using AI in some form, up from about 30% two years ago. The most common applications are data analytics, process automation (like using AI to handle invoices or customer inquiries), and predictive modeling for business forecasts. Notably, Swiss banks and insurers have been investing heavily in AI, but with a cautious approach emphasizing reliability and compliance (for instance, using AI to detect fraud or to streamline customer service, but keeping a human in the loop for final decisions). Swiss manufacturing firms, including precision engineering and pharma companies, are also piloting AI for optimizing production and R&D (such as using machine learning to discover new drug compounds or improve supply chain efficiency in watchmaking). This growing embrace of AI in Switzerland’s traditional industries indicates a recognition that to stay competitive globally, Swiss businesses must leverage advanced technologies. The supportive ecosystem (strong research institutions, a stable regulatory environment, and public-private partnership initiatives like digitalswitzerland) gives Swiss companies a solid foundation to innovate with AI responsibly.
Industry Adoption and Corporate Moves: Swiss industries, especially banking, insurance, and pharmaceuticals, are rapidly adopting AI, often in collaboration with local startups. A great example is the Unique startup mentioned earlier. Founded in Zurich in 2021, Unique transitioned from a sales-tool idea to building an “agentic AI workforce” for finance. In late February it secured $30 million in Series A funding from international investors, aiming to deploy AI agents that handle tedious tasks in banking and insurance – think of due diligence for investments, compliance paperwork, or KYC onboarding. These are areas Swiss financial firms know well (and find costly and time-consuming), so Unique’s AI co-workers offer to boost efficiency and free human employees for higher-level work. That such a sizable investment came in shows confidence in Swiss innovation – and it dovetails with Switzerland’s credibility in finance and trust. We’re likely to see these AI agents trialed at major Swiss banks or insurers soon, given the home-field advantage and connections.
Large Swiss corporates are also making strategic moves. For instance, Zurich Insurance Group (one of the world’s biggest insurers, headquartered in Zurich) just invested in an AI-driven insurtech startup abroad, signaling that Swiss firms want a stake in AI disruption rather than be disrupted themselves. Swiss Life, as noted, is implementing generative AI for client services in partnership with an AI solutions company – a clear sign that even the traditionally conservative insurance sector here recognizes the need to innovate customer experience through AI. In pharmaceuticals, companies like Novartis have been working with AI for drug discovery and clinical trial optimization, often tapping Swiss AI and data startups for collaborations. Swiss manufacturing companies (ABB, for example) are infusing AI into robotics and smart factory systems, capitalizing on Switzerland’s long-standing excellence in precision engineering combined with new AI capabilities.
Breakthrough Research & Innovations (Agentic AI Focus)
The frontier of AI research is brimming with breakthroughs, and a significant theme in recent innovations is the rise of agentic AI – AI systems endowed with a degree of autonomy to perceive, decide, and act in multi-step tasks. This week brought multiple signs that agentic AI is moving from concept to reality:
One major development was Google’s introduction of Agent2Agent (A2A), an open protocol to standardize communication between AI agents. Much like how HTTP enables any web browser to talk to any web server, Google’s A2A (developed with over 50 partner companies) aims to let AI agents from different platforms speak a common language. Why does this matter? As companies deploy various AI agents (one for scheduling, another for inventory management, etc.), a standard would allow these agents to coordinate. For example, a sales agent AI could automatically query a finance agent AI for a client’s credit history, even if they were built by different vendors, as long as both support A2A. This kind of interoperability could unlock complex workflows that today require manual integration. Google’s move indicates that multi-agent systems are becoming mature enough that interoperability standards are needed – a sure sign of this technology’s momentum.
In the startup arena, several new agent platforms launched, betting that businesses are ready for autonomous AI helpers. Writer, known for its AI writing tools, unveiled an “AI HQ” platform that enables companies to deploy custom AI agents for business processes. These agents can execute tasks end-to-end, not just chat – imagine an agent that automatically reads through customer feedback, creates a summary report, and files tickets for any urgent issues it found. Another company, Make (a no-code workflow automation service), rolled out AI Agents for automation. This allows users with no programming background to create agents that make real-time decisions within a workflow. For instance, a Make AI agent could monitor incoming support emails and decide, on its own, whether to send an immediate answer or escalate to a human, based on the email content and past similar cases. And Vurvey Labs introduced an autonomous workflow platform, essentially offering out-of-the-box AI agents tailored to tasks like content moderation or data entry. These releases show a clear trend: delivering AI not just as a model or an API, but as a ready-to-go agent that can be dropped into business processes. It’s a higher-level value proposition – less “here’s a tool, go build with it” and more “here’s a virtual employee you can hire on day one.”
Why the focus on agentic AI? Because it represents the next step in AI’s evolution: from simply providing answers to taking actions. Early evidence of its potential is compelling. OpenAI’s new GPT-4.1 models were explicitly tuned for better “agent” performance – the company noted that GPT-4.1 is more reliable in following through long, complex instructions, which is critical if it’s driving an autonomous process. In tests, these models proved adept at things like reading lengthy documents to extract key info and then using that info to complete a task without human hand-holding. This week, OpenAI also released BrowseComp, a benchmark to test how well AI agents can navigate the web to find information. Essentially, can an AI agent use a browser like a human researcher would? These kinds of benchmarks and improvements are laying the groundwork for AI that doesn’t just sit and answer questions, but actively performs jobs like a junior analyst or assistant might.
Of course, with innovation comes the revealing of limitations. Microsoft Research published a study showing that even the best AI models still struggle with debugging software – the top model solved under 50% of coding bugs in their test, and others were far worse. This suggests that while AI can generate code or do routine tasks well, higher-level problem-solving (especially when it requires reasoning through why something doesn’t work) remains challenging. Similarly, researchers at MIT found that if you feed an AI model irrelevant or misleading context before a question, its performance on tasks like math problems drops significantly – even for very large models. This phenomenon, sometimes called “context poisoning,” implies that prompt design and context management are vital. In an agentic AI scenario, where an agent might gather its own information, ensuring it doesn’t get thrown off by bad data will be a key part of development. These findings are important because they highlight where human oversight and new research are needed: building agents that know when they’re out of their depth or when data might be deceptive.
Microsoft researchers this week also unveiled a project called “BitNet” – an experimental large language model designed to run efficiently on commodity hardware (CPUs) rather than high-end GPUs. BitNet uses a novel weight quantization approach, effectively representing the neural network’s parameters using just three values (-1, 0, +1) instead of full-precision numbers. This radically reduces memory usage and computational needs. The current BitNet model is modest in size (2 billion parameters) but was trained on a hefty dataset (4 trillion tokens) to compensate. Impressively, BitNet achieves performance comparable to larger GPU-dependent models of similar scale, despite being able to run on a standard laptop or even a smartphone chip. It’s also open-source (MIT licensed), inviting developers to experiment. The implications of this are significant: it points toward a future where advanced AI can be embedded in devices or software without cloud dependence, improving privacy and reducing costs. For example, a fintech company could run an AI assistant directly on users’ phones for instant service, or a manufacturer could embed AI in machines on the factory floor for smarter control systems, all without needing an internet connection to a cloud AI. While BitNet is a research prototype, it underscores a broader innovation trend – making AI smaller, faster, and cheaper through clever algorithms. This can democratize AI access beyond big tech data centers, and also help reduce energy consumption of AI workloads (an increasing concern for sustainability).
In the realm of AI alignment and transparency, there was notable progress too. The Allen Institute for AI released OLMoTrace, a tool that lets developers trace which training data snippets most influenced a given output from a language model. In other words, if an AI writes a specific sentence, OLMoTrace can help identify what examples from its training set led to that sentence. For agentic AI systems making decisions, such traceability could be crucial for audits and trust. Imagine an autonomous AI financial advisor – regulators and customers will want to know the basis for its recommendations. Tools like OLMoTrace are early steps toward making AI’s decision-making less of a black box.
On the hardware and performance side, innovations like Google’s Ironwood chip (as mentioned) and other new AI accelerators are enabling much more complex AI workloads. With such hardware, running, say, a hundred AI agents concurrently on live data becomes feasible, where a couple of years ago that would’ve been prohibitively slow or costly. Open-source communities are also contributing: the new DeepCoder-14B model, an open-source 14-billion-parameter AI, was reported to match OpenAI’s proprietary code model on programming tasks despite being smaller. This suggests efficiency gains – through algorithmic improvements or clever training – are still being found. Efficient models that can run on modest hardware will make deploying swarms of AI agents much more accessible to businesses that don’t have Google-level compute resources.
In academic circles, AI is intersecting with neuroscience. A Meta AI researcher gave an interview explaining how neural networks and the human brain might illuminate each other – for instance, larger language models are beginning to show patterns of activity that eerily resemble brain responses in certain language tasks. Understanding this link could inspire new architectures that are more “brain-like” in efficiency and generalization (brains, after all, run on about 20 watts of power – far less than those data centers). It’s not directly “agentic AI,” but it all feeds into creating AI that can learn and adapt more autonomously like a human.
Bringing it back to agentic AI: the idea of autonomous AI agents has captured the imagination because it promises to automate not just labor, but autonomy itself. Instead of us micromanaging software, we could delegate objectives and let AI figure out the rest. The flurry of protocols, platforms, and research this week shows that many smart minds are working to overcome the challenges – interoperability, reliability, safety, transparency – that stand between us and truly useful AI agents. It’s a fast-moving frontier. For businesses, agentic AI could mean an explosion of productivity: routine multi-step workflows handled start-to-finish by tireless assistants. It could also raise new questions: how do we govern AI “employees”? How do we integrate them into teams? What new skills will our human staff need (perhaps “agent management” will be a role, analogous to managing human teams)? Those conversations are just beginning, but it’s notable that 2025 is shaping up to be the year agentic AI steps onto the main stage.
Conclusion
The week of April 14–20, 2025, offered a snapshot of an AI landscape that is evolving at breakneck speed.
The concept of agentic AI underscores how far the field may go: we’re talking about AI that can act on your behalf, not just answer questions. This could transform white-collar work and beyond. Companies that start pilot projects with AI agents – even something as simple as an agent to automate internal IT support tickets – will learn valuable lessons now and position themselves to benefit as the technology matures. It’s also prudent to establish internal AI guidelines (for example, a policy on validating AI-generated content or decisions) to ensure that as you scale up AI usage, it remains aligned with your business standards and customer expectations.
Another takeaway is the importance of data – the fuel of AI. With giant models like GPT-4.1 now able to consume massive context, organizations that have their data in order (clean, well-structured, and accessible to feed into AI) will get more out of these tools. Conversely, issues like the Meta lawsuit remind us that using data without clear rights can backfire. So, an investment in robust data governance and perhaps synthetic data generation (to augment datasets ethically) can pay dividends.
The landscape can be dizzying, but one thing is certain: the AI era is here, and it’s advancing weekly. By proactively engaging with these trends – as seen in the use cases, vendor innovations, governance shifts, and research breakthroughs of this week – business leaders can ensure they ride the AI wave rather than be drowned by it. In Switzerland and beyond, the organizations that blend technological prowess with responsible practices will likely lead the pack in this exciting new chapter of the digital age.
Sources:
OpenAI News: “Introducing GPT-4.1 in the API” – OpenAI official blog (April 2025).
TechCrunch: “OpenAI plans to phase out GPT-4.5, recommends switch to GPT-4.1” (April 14, 2025).
TechCrunch: “ChatGPT became the most downloaded app globally in March” (April 11, 2025).
OpenAI News: “BrowseComp: Benchmarking AI web browsing” – OpenAI release (April 2025).
The Decoder: “ChatGPT’s expanded memory raises privacy eyebrows” (April 2025).
TechCrunch: “OpenAI launches program for domain-specific AI benchmarks” (April 2025).
TechCrunch: “OpenAI countersues Elon Musk amid legal battle” (April 2025).
Google Blog: “New AI features in Google Workspace apps” (April 2025).
Google Blog: “Expanding Google Agentspace for easy AI agent creation” (April 2025).
VentureBeat: “Google introduces Firebase Studio for no-code app development” (April 9, 2025).
VentureBeat: “Google launches Gemini AI coding assistant for enterprise” (April 9, 2025).
VentureBeat: “Google’s new Ironwood chip is 24× faster than the top supercomputer” (April 9, 2025).
Azure Blog: “Microsoft brings GPT-4.1 models to Azure OpenAI Service” (April 15, 2025).
The Decoder: “Microsoft adjusts AI infrastructure plans, pauses Ohio data centers” (April 2025).
TechCrunch: “Meta’s ‘Maverick’ AI model underperforms on benchmark after controversy” (April 2025).
TechCrunch: “Meta to train AI on EU public data with user opt-out after regulatory clarity” (April 2025).
The Verge: “FTC vs. Meta: Antitrust trial begins – what’s at stake” (April 14, 2025).
TechCrunch: “Law professors back authors in suit over Meta’s AI training data” (April 16, 2025).
Forbes: “U.S. AI policy pivots from safety to security focus” (April 15, 2025).
The Decoder: “Irish DPC investigates X’s use of data for Grok AI” (April 2025).
Reuters: “EU plans €200 billion ‘CERN for AI’ research initiative; Switzerland’s participation uncertain” (April 2, 2025).
Swissinfo: “Switzerland risks exclusion from Europe’s ‘CERN for AI’” (April 2, 2025).
United Nations Report: “Global AI Readiness Index 2025” – UN AI & Equality report (April 2025).
Swiss Federal Council Press Release: “Federal Council presents approach on AI – adopting Council of Europe Convention” (Feb 13, 2025).
ETH Zurich News: “Strengthening Switzerland as an AI hub” (ETH press release, April 15, 2025).
Fintech News Switzerland: “Unique (Zurich) secures $30M to pioneer agentic AI in finance” (Feb 27, 2025).
TechCrunch: “Unique, a Swiss AI platform for finance, raises $30M” (Feb 27, 2025).
GlobeNewswire: “BEN and Swiss Life launch AI partnership for insurance solutions” (April 1, 2025).
TechCrunch: “Zurich Insurance invests in AI insurtech Ominimo at €220M valuation” (April 14, 2025).
VentureBeat: “Google’s Agent2Agent protocol to standardize AI agent communication” (April 9, 2025).
AIthority: “Writer unveils ‘AI HQ’ for autonomous business agents” (April 2025).
AIthority: “Make introduces AI Agents for no-code workflow automation” (April 2025).
TechCrunch: “Anthropic launches $200/month Claude Max for power users” (April 10, 2025).
TechCrunch: “Google to embrace Anthropic’s model-context standard for AI” (April 9, 2025).
TechCrunch: “xAI opens API for Grok model as questions about neutrality emerge” (April 2025).
The Decoder: “xAI alters Grok to deflect misinformation claims involving Musk” (April 2025).
The Decoder: “Alibaba’s Quark now China’s top AI app with 150M users” (Apr 14, 2025).
VentureBeat: “ByteDance unveils reasoning-focused AI model Seed-Thinking-v1.5” (April 8, 2025).
Reuters: “Baidu to open-source its Ernie AI model by June 30” (Feb 14, 2025).
Forbes: “AI pricing faces pressure beyond the $20/month era” (April 17, 2025).
Forbes: “The rise of ‘soft AI’ and what it means for businesses” (April 18, 2025).
TechCrunch: “Amazon CEO Jassy: companies should invest heavily in AI now” (April 15, 2025).
TechCrunch: “Benchmarking ‘reasoning’ AI models is getting very expensive” (April 15, 2025).
TechCrunch: “Microsoft study: AI models struggle with debugging tasks” (April 16, 2025).
The Decoder: “One in five AI-generated code suggestions references fake libraries (new security risk)” (April 2025).
TechCrunch: “MIT study finds AI models don’t have consistent ‘values’” (April 14, 2025).
The Decoder: “MIT: Irrelevant input can cause large errors in LLMs’ outputs” (April 2025).
The Decoder: “Allen Institute’s OLMoTrace tool can trace LLM outputs back to training data” (April 2025).
Meta AI Interview: “Neuroscience meets AI – researcher on brain and AI similarities” (April 2025, The Decoder).
TechCrunch: “Salesforce developers embrace AI coding tools as roles evolve” (April 13, 2025).
TechCrunch: “Startup Artisan raises $25M for AI sales agents, reflects on lessons learned” (April 14, 2025).
TechCrunch: “Incident.io raises $62M for AI-driven incident response platform” (April 15, 2025).